Dr. Dongeun Lee
Challenges in Statistical Similarity Based Data Reduction
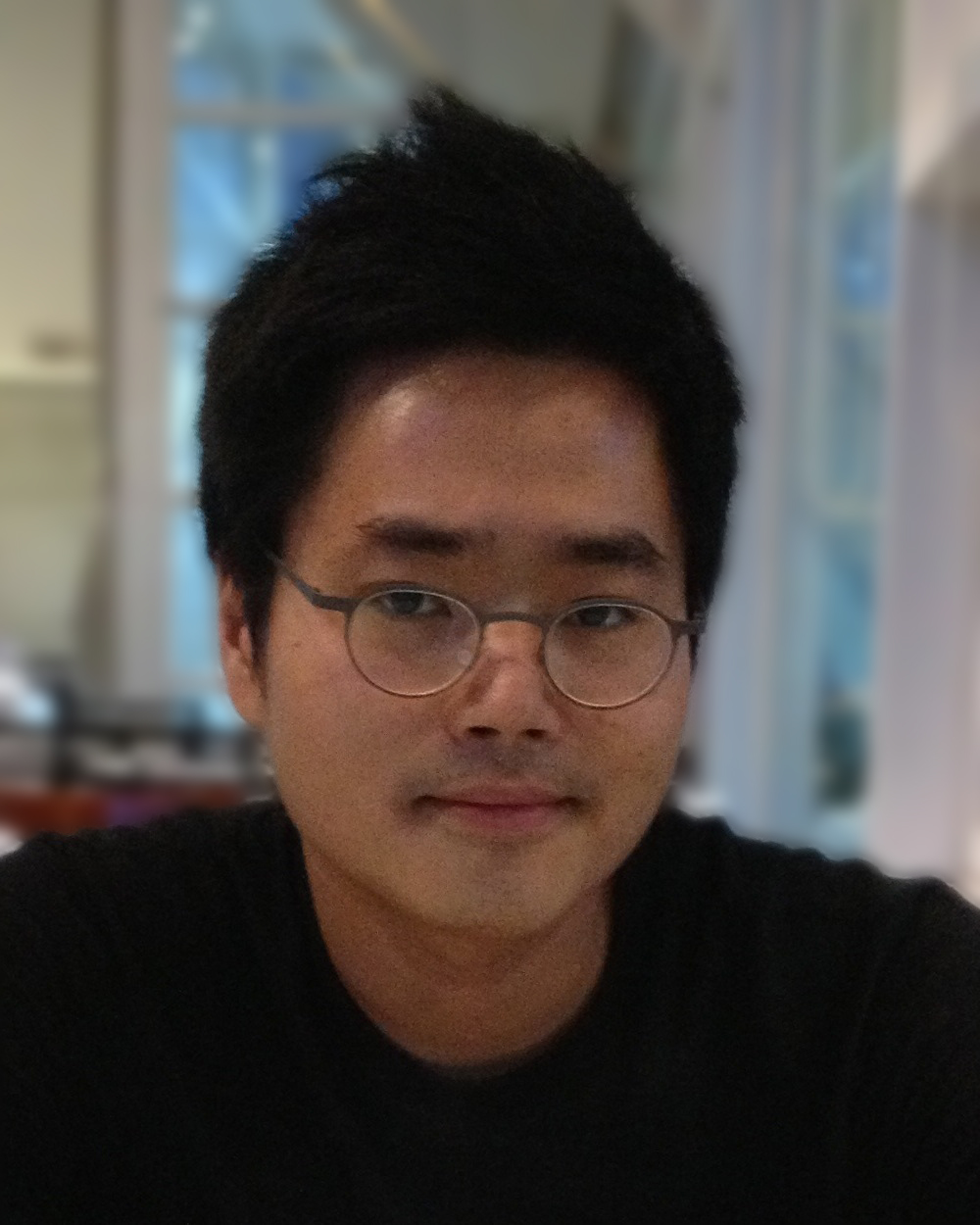
|
Speaker:
Dongeun Lee, PH.D
Assistant Professor
Date: Thu, 11.29.2018
Time: 4:30-5:30pm
Journalism Bldg-Room:129
Host: Dr. Abdullah Arslan
|
Big data applications are generating so much data quickly that compression is essential to reduce storage requirement or transmission capacity. Most existing data compression methods rely on Euclidean distance measure to discard redundant information, which severely limits compression performance. When applied to big data, they may produce summaries of data which are still in high volume but fail to capture important characteristics of dataset a domain expert would otherwise recognize and extract. The recently introduced statistical similarity based data reduction showed great potential to address this issue. However, there are still challenges for fully leveraging the potential of this new approach. This talk will introduce the statistical similarity based data reduction and its potential for many big data applications, followed by the discussion of challenges and future research directions.